Container vulnerability scanning leaves security teams in a tough place. On the one hand, some argue that container scanning has been commoditized due to numerous open source projects that support vulnerability scanning as a feature. On the other hand, they’re so annoying to deal with that companies like Chainguard can succeed just by saying they make them go away (the truth is more complicated).
The confusion stems from a core reality: finding vulnerabilities is a million times easier than fixing them. In this article, we’ll discuss why container vulnerabilities are so hard to fix, before addressing the critical information needed to prioritize and remediate them.
Why Container Vulnerabilities are an Issue
When we talk about container vulnerabilities, we’re mostly talking about operating system vulnerabilities, but people don’t treat them that way. Building a container is like building a virtual machine. You take your golden image, do some customization to it, and deploy it out to production. Unlike Windows operating systems, once an image is deployed, there’s typically not any “auto-updating” done, which is what creates vulnerabilities to be remediated.
This means two things:
- It’s not always clear where a vulnerable package is coming from
- The context of how you use that package is usually not exploitable
Overall, developers get really frustrated because they’re asked to fix findings which are not exploitable, and it’s unclear what to even do to fix them. Do I need a new base image? Do I need to change a line of code? Do I need to just redeploy?
Without more information about the container itself, how it’s built, and the context of its deployment, vulnerability scanning leads to a never ending trail of unfixable and unexploitable findings.
How to Prioritize
These days, every solution is offering the silver bullet to “risk based prioritization,” but without runtime Kubernetes context, many vendors are relying solely on vulnerability data to try and create their prioritization. Security teams need contextual data points that go beyond KEV and EPSS.
In general, there are three vulnerability prioritization methods at work in containers:
- Is the package in use?
- Is there an attack path to the package from the internet?
- How many workloads are running the vulnerability?
First, many container images contain packages that aren’t used by the container when it’s running. In this article, I’m talking about in-use only in terms of the package being loaded into memory, but in the future I’ll break down other meanings that make even this idea hard to dissect. These unused packages are left over artifacts either from operating system utilities or dependencies used only for building or testing images. To put it succinctly, if a dependency isn’t running, then it can’t be part of an exploit unless an attacker is already inside a system, making it a lower priority.
Second, while patching is still important for defense in depth, most exploits happen when packages are publicly facing. These are the critical zero days security teams worry about patching, because it’s a guarantee that end users can hit the endpoints. I’ve written elsewhere about the pros and cons of network reachability – but in sum it’s an important data point, not a silver bullet.
Finally, deciding what vulnerabilities to patch also comes down to a realistic “bang for your buck” approach. Developer time is limited, so if you’re prioritizing which of thousands of vulnerabilities to tackle, it makes sense to patch the images that are most widely distributed to most significantly reduce your attack surface.
A quick word on static reachability like what Endor, Backslash, Aikido, and others provide – this data is especially applicable for code library vulnerabilities, where you can look at the code to try and tell if a vulnerable function is getting called by your code. This logic is less applicable to containers though, because system OS libraries require at least some sort of simulation to tell if they’re loading or not. Even on the SCA side, in my testing, there is a real trade off between when you’re scanning and the accuracy of the de-prioritization. For example, in my test repo, I have some scripts that aren’t actually used anywhere – only runtime tools can tell they’re not used.
On their own, these three prioritization data points hold limited value, but when put together security teams are able to drive an effective prioritization program. For example, internet facing has a wide variety of meanings, so while it’s useful as a data point for vulnerability prioritization, it needs additional context to fully prioritize. In sum, the more data the better – as business needs will constantly fluctuate, causing different prioritizations along the way.
How to Fix
Once a developer gets a prioritized, fixable ticket they can action, there are three possible actions that might happen:
- Simply re-building the container inherits all available patches
- They need to swap or upgrade base images
- They need to change a line of code in the Dockerfile to upgrade a package
To make this decision, tools can help by providing some key information. First, developers need to know what layer of the image the vulnerability came in from. Take this simple example from a node-js base image, node:16
In this image, I use the base Nodejs image, and then npm install my packages. Let’s say I get a vulnerability finding: high vulnerability with lodash
Without more information, I’m dead in the water trying to figure out where this is coming from. Unless I’ve memorized every dependency, direct and transitive, I’m going to have to start researching possible ways this got into my image. But if I have the layer information:
I can see that it comes in when I run npm install, telling me this is an npm dependency I need to go and update. Similarly, if it’s a critical enough vulnerability, the image hierarchy at the top tells me how the base node image itself is constructed, telling me exactly what is and isn’t in my control to fix.
Layer information is so critical I’d struggle to use any tool that didn’t have it, and the few times I’ve been forced to have been monumental struggles. In the above example, I can at least see where a vulnerability is coming from – whether a base image, an ubuntu package I’m installing, or my npm install. Without this information, I’d just be dead in the water trying to hunt down how to fix something.
Second, it’s important that scanners offer multiple places to scan so I can quickly validate my findings. Let’s say I suspect a simple rebuild will fix my issues, the first thing I’ll do is build the container locally. If I have to first push that container all the way out to production, it creates a painful feedback loop to try and see if I’ve fixed the issue. I’d love for a tool to be able to detect if a rebuild would fix the vulnerability, as most developers I’ve worked with don’t realize this is all that’s needed to fix a finding.
Finally, if I need to upgrade base images, it’s helpful if the tool lets me know what versions of the image are available, and what the vulnerability statuses on those versions are. It’s often the case that upgrading versions can actually increase vulnerability counts. Note: base images are often patched without changing version numbers!
Overall, the problems with container vulnerability scanning are twofold: not enough data to prioritize, and not enough data to fix. On the prioritization side, a lot of contextual information is needed to drive effective prioritization, and to be honest every company will choose slightly different metrics based on their specific application. In general, some kind of contextual, runtime data is needed on this side.
On the fix side, it’s important to have the image layers, fix versions, and upgrade paths available. The thing that would be most helpful but no one has yet is just letting me know if a simple rebuild would fix a vulnerability as opposed to needing to manually change anything!
Tools for container vulnerability scanning
Tools for vulnerability management and contextualization
This post was completed in collaboration with ARMO
转自:https://pulse.latio.tech/p/how-container-vulns-get-fixed
google翻译:
容器漏洞扫描让安全团队陷入困境。一方面,有人认为容器扫描已经商品化,因为许多开源项目都支持漏洞扫描功能。另一方面,它们太烦人了,以至于像 Chainguard 这样的公司只要说他们让它们消失就可以成功(事实要复杂得多)。
这种困惑源于一个核心现实:发现漏洞比修复漏洞容易一百万倍。在本文中,我们将讨论为什么容器漏洞如此难以修复,然后介绍确定优先级并修复漏洞所需的关键信息。
容器漏洞为何是一个问题
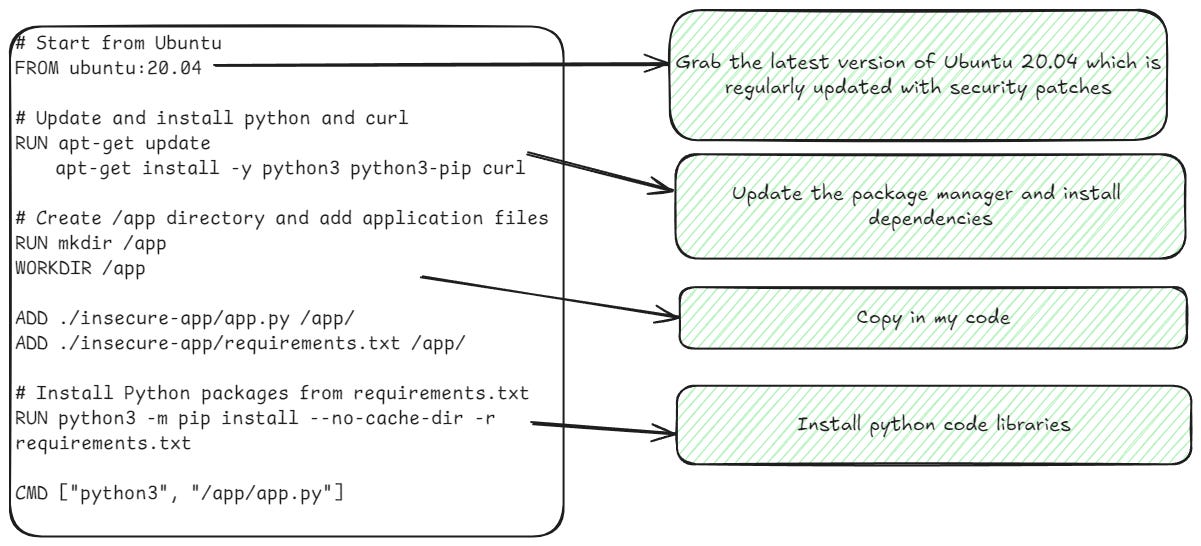
当我们谈论容器漏洞时,我们主要谈论的是操作系统漏洞,但人们并不这样看待它们。构建容器就像构建虚拟机。你获取黄金映像,对其进行一些自定义,然后将其部署到生产中。与 Windows 操作系统不同,一旦部署映像,通常不会进行任何“自动更新”,而这正是造成漏洞需要修复的原因。
这意味着两件事:
- 存在漏洞的软件包来自哪里并不总是很清楚
- 您使用该包的上下文通常是不可利用的
总的来说,开发人员真的很沮丧,因为他们被要求修复无法利用的漏洞,而且他们甚至不清楚该怎么做才能修复它们。我需要一个新的基础镜像吗?我需要更改一行代码吗?我只需要重新部署吗?
如果没有关于容器本身、容器如何构建以及容器部署环境的更多信息,漏洞扫描将导致无法修复和无法利用的结果。
如何确定优先顺序
如今,每种解决方案都提供了“基于风险的优先级排序”的灵丹妙药,但由于没有运行时 Kubernetes 上下文,许多供应商只能依靠漏洞数据来尝试创建优先级排序。安全团队需要超越 KEV 和 EPSS 的上下文数据点。
一般来说,容器中有三种漏洞优先级排序方法:
- 该包是否正在使用中?
- 是否存在来自互联网的攻击路径?
- 有多少工作负载正在运行该漏洞?

首先,许多容器镜像包含容器运行时未使用的包。在本文中,我仅从包加载到内存的角度来讨论“正在使用”,但将来我会分解其他含义,这些含义甚至使这个想法也难以剖析。这些未使用的包是操作系统实用程序或仅用于构建或测试镜像的依赖项的遗留产物。简而言之,如果依赖项未运行,那么它就不能成为漏洞利用的一部分,除非攻击者已经进入系统,因此优先级较低。
其次,虽然修补对于纵深防御仍然很重要,但大多数漏洞都是在软件包公开时发生的。这些是安全团队担心修补的关键零日漏洞,因为它是最终用户可以访问端点的保证。我曾在其他地方写过关于网络可达性的利弊 – 但总而言之,这是一个重要的数据点,而不是灵丹妙药。
最后,决定修补哪些漏洞也取决于现实的“物有所值”方法。开发人员的时间有限,因此如果您要优先解决数千个漏洞中的哪些,那么修补分布最广泛的图像是有意义的,这样可以最大程度地减少您的攻击面。
简单说一下Endor 、Backslash 、Aikido和其他公司提供的静态可达性 – 这些数据特别适用于代码库漏洞,您可以查看代码以尝试判断代码是否调用了易受攻击的函数。但是,这种逻辑不太适用于容器,因为系统操作系统库至少需要某种模拟才能判断它们是否正在加载。即使在 SCA 方面,在我的测试中,扫描时间和降级准确性之间也存在真正的权衡。例如,在我的测试存储库中,我有一些脚本实际上没有在任何地方使用 – 只有运行时工具才能判断它们没有被使用。
就其本身而言,这三个优先级数据点的价值有限,但当它们组合在一起时,安全团队能够推动有效的优先级计划。例如,面向互联网具有多种含义,因此虽然它可用作漏洞优先级的数据点,但需要额外的背景信息才能完全确定优先级。总之,数据越多越好 – 因为业务需求会不断波动,从而导致优先级的差异。
如何修复
一旦开发人员获得可采取行动的优先且可修复的票据,可能会发生三种可能的操作:
- 只需重新构建容器即可继承所有可用的补丁
- 他们需要交换或升级基础镜像
- 他们需要更改 Dockerfile 中的一行代码来升级软件包
为了做出这一决定,工具可以通过提供一些关键信息来提供帮助。首先,开发人员需要知道漏洞来自镜像的哪一层。以 node-js 基础镜像 node:16 中的这个简单示例为例
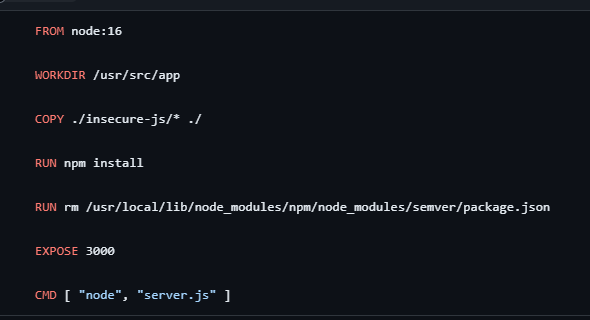
在此镜像中,我使用基本 Nodejs 镜像,然后 npm 安装我的软件包。假设我得到一个漏洞发现:lodash 的高漏洞

如果没有更多信息,我就会陷入困境,无法弄清楚这是从哪里来的。除非我记住了每一个依赖关系,无论是直接的还是传递的,否则我将不得不开始研究这种依赖关系进入我的图像的可能方式。但如果我有层信息:
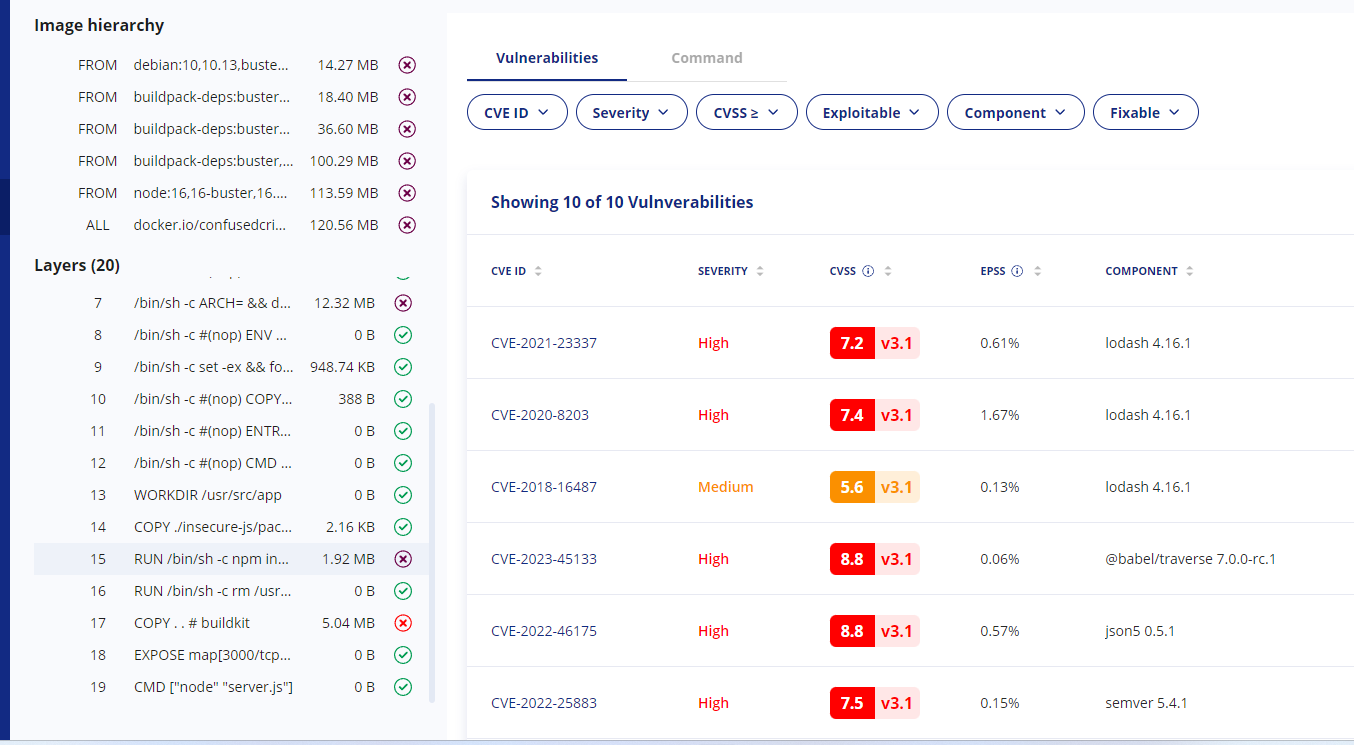
当我运行 npm install 时,我可以看到它出现,告诉我这是一个需要更新的 npm 依赖项。同样,如果这是一个足够严重的漏洞,顶部的镜像层次结构会告诉我基础节点镜像本身是如何构建的,告诉我哪些是我可以修复的,哪些不是我可以修复的。
层信息如此重要,以至于我很难使用任何没有这些信息的工具,而且有几次我不得不这样做,这真是一场巨大的斗争。在上面的例子中,我至少可以看到漏洞来自哪里——无论是基础映像、我正在安装的 ubuntu 包,还是我的 npm 安装。如果没有这些信息,我就会陷入困境,无法找到修复方法。
其次,扫描仪提供多个扫描位置很重要,这样我就可以快速验证我的发现。假设我怀疑简单的重建可以解决我的问题,我要做的第一件事就是在本地构建容器。如果我必须先将该容器推向生产环境,就会产生一个痛苦的反馈循环,以尝试查看我是否已解决问题。我希望有一种工具能够检测重建是否能修复漏洞,因为我合作过的大多数开发人员都没有意识到这是修复发现所需的全部操作。
最后,如果我需要升级基础映像,如果该工具让我知道映像有哪些版本可用,以及这些版本的漏洞状态如何,那就很有帮助了。通常情况下,升级版本实际上会增加漏洞数量。注意:基础映像通常会在不更改版本号的情况下进行修补!
总体而言,容器漏洞扫描存在两个问题:没有足够的数据来确定优先级,也没有足够的数据来修复。在优先级方面,需要大量的上下文信息来推动有效的优先级排序,老实说,每家公司都会根据其具体应用选择略有不同的指标。一般来说,这方面需要某种上下文运行时数据。
在修复方面,重要的是要有可用的映像层、修复版本和升级路径。最有帮助但还没有人知道的是,只是让我知道简单的重建是否可以修复漏洞,而不是需要手动更改任何内容!
这篇文章是与ARMO合作完成的
转载请注明:jinglingshu的博客 » How Container Vulns Get Fixed–修复容器漏洞的流程